ADM-G¶
This document is devoted to the resolution of discrete mean field games via ADM-G, see (see [HTY12] for a presentation). In short ADM-G consists in an ADMM step followd by a gaussian back substitution step. Compare to ADMM, theorical convergence results are available even for a large number of successive minimization variables.
Packages¶
import numpy as np
from numpy import random
from mpl_toolkits import mplot3d
import matplotlib
import matplotlib.pyplot as plt
import matplotlib.animation as animation
import time
Potential formulation and dual minimization¶
We define a dual criterion
and a dual problem
where
and
Then we define the Lagrangian associated to the above problem:
We define the Augmented Lagrangian parametrized by \(r>0\) as follows
Algorithm¶
Preliminary computations
Let
We have that
Then for any \(v = (\gamma,P,a,b,m,w)\) we have that
The Algorithm is given by :
\((u^0,\gamma^0,P^0,a^0,b^0,m^0,w^0) \leftarrow (u,\gamma,P,a,b,m,w)\)
For \(0\leq n < N\)
Step 1 : ADMM steps
Find
Find
Find
Actualise \((m,w)\) via a gradient descent step
Step 2 : Gaussian Back substitutions
Let \(v^n = (\gamma^{n},P^{n},a^{n},b^{n},m^{n},w^{n})\) and \(\xi \in (0,1)\). Then actualise
End for
Return \((u^N,\gamma^N,P^N,a^N,b^N,m^N,w^N)\).
Data of the problem¶
def initial_mass(n):
m_bar = np.zeros(n)
for x in range(n):
m_bar[x] = np.exp(-(x-n/2)**2/(n/4)**2)
return(n*m_bar/np.sum(m_bar))
def displacement_cost(T,n):
dx=1/n
dt=1/T
disp = np.zeros((T-1,n,n))
for t in range(T-1):
for x in range(n):
for y in range(n):
disp[t,x,y] = ((y-x)*dx/dt)**2/4
return(disp)
def penalisation_congestion(T,n):
nu = np.zeros((T,n))
for t in range(T):
if t > T/2 and t < 3*T/4:
for x in range(n):
if x > n/2:
nu[t,x] = 10
return(nu)
def sharp_penalisation_congestion(T,n):
eta = np.zeros((T,n)) + 3
for t in range(T):
for x in range(n):
if (t > T/3 and t < 2*T/3) and (x > n/3 and x < 2*n/3):
eta[t,x] = 1/4
return(eta)
def reference_demand(T):
new_Db = np.zeros(T-1)
for t in range(T-1):
new_Db[t] = np.sin(t*(4*np.pi)/(T-1))
return(new_Db)
def alpha_cost(T,n):
dx=1/n
dt=1/T
disp = np.zeros((T-1,n,n))
for t in range(T-1):
for x in range(n):
for y in range(n):
disp[t,x,y] = (y-x)*dx/dt
return(disp)
def alpha_bar(alpha,T,n):
new_alpha = np.zeros((T-1,n))
a = np.zeros(T-1)
for t in range(T-1):
for x in range(n):
new_alpha[t,x] = np.sum(alpha[t,x]**2)
a[t] = np.sum(new_alpha[t])
return(a)
Computation of ADMM steps¶
Computation of \(P^{n+1}\)¶
Proximal formulation
where the division has to be understood as a Hadamard product and
Then \(P^{n+1}\) is given by
Computation via Moreau’s identity:
Due to the above definition of \(\phi^\star\) and the Moreau identity, we have that
for any \(\lambda>0\). In addtion for any \(\xi>0\) we have that
def prox_phi_quad(X,Db,D_0,tau,D_min,D_max):
argmin = (X - tau*D_0*Db)/(1 + tau*D_0)
if argmin > D_max:
argmin = D_max
if argmin < D_min:
argmin = D_min
return(argmin)
def Local_P_prox_quad(u,b,w,n,r,alpha,var_alpha,Db,D_0,D_min,D_max,t):
dt=1/T
dx=1/n
Z = np.zeros((n,n))
for x in range(n):
Z[x] = (w[t,x]/r - u[t+1]/dt - b[t,x])*alpha[t,x]
X = np.sum(Z)/var_alpha[t]
lambde = 1/(r*var_alpha[t]*dx)
new_Pt = X - lambde*prox_phi_quad(X/lambde,Db[t],D_0,1/lambde,D_min,D_max)
return(new_Pt)
def P_argmin_L(u,b,w,T,n,r,alpha,var_alpha,Db,D_0,D_min,D_max):
new_P = np.zeros(T-1)
for t in range(T-1):
new_P[t] = Local_P_prox_quad(u,b,w,n,r,alpha,var_alpha,Db,D_0,D_min,D_max,t)
return new_P
Computation of \(u^{n+1}\)¶
\(u^{n+1}\) is given by
with
Then
for any \(t \in \bar{\mathcal{T}}\).
def u_argmin_L(P,gamma,a,b,m,w,m_0,alpha,T,n,r):
dt=1/T
new_u = np.zeros((T,n))
for t in range(T):
for x in range(n):
if t==0:
new_u[t,x] = ((m_0[x] - m[t,x])/r + gamma[t,x] + a[t,x])*dt
elif t==(T-1):
new_u[t,x] = ((-m[t,x] + np.sum(w[t-1,:,x]))/r + gamma[t,x] - np.sum(alpha[t-1,:,x]*P[t-1] + b[t-1,:,x]))/(1+n/dt)
else:
new_u[t,x] = ((-m[t,x] + np.sum(w[t-1,:,x]))/r + gamma[t,x] + a[t,x] - np.sum(alpha[t-1,:,x]*P[t-1] + b[t-1,:,x]))*dt/(1+n)
return new_u
Computation of \(\gamma^{n+1}\)¶
Proximal formulation
\(\gamma^{n+1}\) is given by
for any \(t \in \bar{\mathcal{T}}\).
Computation via Moreau’s identity:
Due to the above definition of \(F^\star\) and the Moreau identity, we have that
for any \(\lambda>0\). In addtion for any \(\xi>0\) we have that
def prox_coeff_F_sharp(X,nu,eta,coeff):
argmin = (X - coeff*nu)/(coeff + 1)
if argmin>eta:
argmin = eta
if argmin<0:
argmin = 0
return(argmin)
def Moreau_id_F(X,nu,eta,lambde):
return(X-lambde*prox_coeff_F_sharp(X/lambde,nu,eta,1/lambde))
def Moreau_gamma_argmin_L(u,a,m,nu,eta,T,n,r):
dt = 1/T
new_gamma = np.zeros((T,n))
for t in range(T):
for x in range(n):
if t == T-1:
X = m[t,x]/r + u[t,x]
new_gamma[t,x] = Moreau_id_F(X,nu[t,x],eta[t,x],1/r)
else:
X = m[t,x]/r + u[t,x]/dt - a[t,x]
new_gamma[t,x] = Moreau_id_F(X,nu[t,x],eta[t,x],1/r)
return(new_gamma)
Computation of \((a^{n+1},b^{n+1})\)¶
In the linear case, the running cost is given by
with dom\((\ell(t,x,\rho)) = \Delta(S)\). Thus the set \(\mathcal{Q}\) is given by
We define
Then for any \((t,x) \in \mathcal{T} \times S\), we aim at finding
where
To compute this projection, we define the following minimisation problem
The solution to the subproblem
is given by
Thus replacing in the initial problem we now need to solve the following optimization problem
Let \(\tilde{\beta}(y) := \beta(y)-\bar{b}(y)\). We denote \((y_k)_{k\in \{0,\ldots,n-1\}}\) the sequence such that
Then the optimal \(a\) is such that
Optimal a
We have that
Then we define the index \(k \in \{1,\ldots,n-1 \}\) if the following conditions are satisfied
Otherwise we set \(k = n\) if \( \partial g (\tilde{\beta}(y_{n-1})) \leq 0\) and \(k = 0\) if \( \partial g (\tilde{\beta}(y_{0})) \geq 0\).
We finally deduce that
Optimal b
def proj_Q(a_bar,b_bar,beta,n):
a = 0
b = np.zeros(n)
beta_bar = beta-b_bar
beta_bar_sort = np.sort(beta_bar)
k = 0
sum_beta_bar_sort = 0
derivative_beta = (k+1)*beta_bar_sort[k] - a_bar - sum_beta_bar_sort
while (derivative_beta < 0) and (k < n-1):
sum_beta_bar_sort = sum_beta_bar_sort + beta_bar_sort[k]
k = k+1
derivative_beta = (k+1)*beta_bar_sort[k] - a_bar - sum_beta_bar_sort
if (k==n-1) and (derivative_beta < 0):
sum_beta_bar_sort = sum_beta_bar_sort + beta_bar_sort[k]
k = n
a = (a_bar + sum_beta_bar_sort)/(k+1)
for y in range(n):
b[y] = np.min(np.array([b_bar[y],beta[y] - a]))
return (a,b)
def a_b_proj(P,gamma,u,m,w,T,n,r,alpha,beta):
dt=1/T
(new_a,new_b) = (np.zeros((T-1,n)),np.zeros((T-1,n,n)))
for t in range(T-1):
for x in range(n):
c_1 = m[t,x]/r + u[t,x]/dt - gamma[t,x]
c_3 = w[t,x]/r - alpha[t,x]*P[t] - u[t+1]/dt
(new_a[t,x],new_b[t,x]) = proj_Q(c_1,c_3,beta[t,x],n)
return(new_a,new_b)
#print(proj_Q(np.array([2]),np.array([2,2,-2,5,3]),np.array([0,1,2,3,3]),5))
Computation of \((m^{n+1},w^{n+1})\)¶
We recall that the actualisation of \((m^{n+1},w^{n+1})\) is given by a gradient descent step
def m_w_gradient_step(P,gamma,u,a,b,m,w,q,T,n):
dt=1/T
new_m = np.zeros((T,n))
new_w = np.zeros((T-1,n,n))
for t in range(T-1):
for x in range(n):
new_m[t,x] = m[t,x] + q*(u[t,x]/dt - gamma[t,x] - a[t,x])
new_w[t,x] = w[t,x] + q*(- alpha[t,x]*P[t] - u[t+1]/dt - b[t,x])
new_m[T-1] = m[T-1] + q*(u[T-1] - gamma[T-1])
return (new_m,new_w)
Strategy \(\pi = w/m\) and average strategy
def equilibrium_strategy(m,w,T,n,tol=1e-5):
strat = np.zeros((T-1,n,n))
for t in range(T-1):
for x in range(n):
if m[t,x] > tol:
strat[t,x] = w[t,x]/m[t,x]
return(strat)
def mean_field_strategy(pi,T,n):
mfs = np.zeros((T-1,n))
for t in range(T-1):
for x in range(n):
for y in range(n):
mfs[t,x] += pi[t,x,y]*(y-x)
return(mfs)
Gaussian Back step¶
Let \(\xi \in (0,1)\),
def GB_step(gamma,P,a,b,m,w,tilde_u,tilde_gamma,tilde_P,tilde_a,tilde_b,tilde_m,tilde_w,xi,alpha,var_alpha,mfg=1):
new_P = np.zeros(T-1)
new_a = a + xi*(tilde_a - a)
new_b = b + xi*(tilde_b - b)
new_m = m + xi*(tilde_m - m)
new_w = w + xi*(tilde_w - w)
new_gamma = gamma + xi*(tilde_gamma - gamma - (tilde_a - a))
if mfg == 0:
for t in range(T-1):
new_P[t] = P[t] + xi*(tilde_P[t] - P[t] - np.sum(alpha[t]*(tilde_b[t] - b[t])/var_alpha[t]))
new_u = tilde_u
return(new_u,new_gamma,new_P,new_a,new_b,new_m,new_w)
Reconstruction of \((u,\pi[u])\)
def d_p_mapping(m,w,gamma,P,alpha,beta,T,n,tol = 1e-3,mfg=1):
dt=1/T
dx=1/n
new_u = np.zeros((T,n))
new_pi = np.zeros((T-1,n,n))
f = np.zeros((T,n))
if mfg == 1:
f = gamma
new_u[T-1] = f[T-1]
for t in range(T-1):
for x in range(n):
cost = np.zeros(n)
cost = beta[T-2-t,x]*dt + alpha[T-2-t,x]*P[T-2-t]*dt + new_u[T-1-t]
argmin = np.argmin(cost)
if m[T-2-t,x]*dx>tol:
new_pi[T-2-t,x] = w[T-2-t,x]/m[T-2-t,x]
else:
new_pi[T-2-t,x,argmin] = 1
new_u[T-2-t,x] = cost[argmin] + f[T-2-t,x]*dt
return(new_u,new_pi)
Errors¶
def verification(P,u,gamma,m,pi,w,T,n,beta,nu,m_0,alpha,Db,Dmin,Dmax,eta,mfg = 1):
cost = np.zeros((T-1,n))
Delta_u = np.zeros((T,n))
Delta_pi = np.zeros((T-1,n,n))
Delta_m = np.zeros((T,n))
Delta_gamma = np.zeros((T,n))
Delta_P = np.zeros(T-1)
demand = np.zeros((T-1,n,n))
dt=1/T
dx=1/n
f = np.zeros((T,n))
if mfg == 1:
f = gamma
Delta_m[0] = abs(m[0] - m_0)*dx
for x in range(n):
Delta_u[T-1,x] = abs(u[T-1,x] - f[T-1,x])*dx
for t in range(T-1):
for x in range(n):
arg = beta[t,x]*dt + alpha[t,x]*P[t]*dt + u[t+1]
demand[t,x] = pi[t,x]*m[t,x]*alpha[t,x]*dx
cost[t,x] = np.sum(pi[t,x]*(beta[t,x]*dt + alpha[t,x]*P[t]*dt)) + f[t,x]*dt
Delta_pi[t,x] = abs(np.sum(pi[t,x]*arg) - np.sort(arg)[0])*dx
Delta_m[t+1,x] = abs(m[t+1,x] - np.sum(pi[t,:,x]*m[t]))*dx
Delta_u[t,x] = abs(u[t,x] - cost[t,x] - np.sum(pi[t,x]*u[t+1]))*dx
if gamma[t,x] < nu[t,x]:
Delta_gamma[t,x] = abs(gamma[t,x])*dt*dx
elif gamma[t,x] > eta[t,x] + nu[t,x]:
Delta_gamma[t,x] = abs(gamma[t,x] - eta[t,x])*dt*dx
else:
Delta_gamma[t,x] = abs(gamma[t,x] - m[t,x] - nu[t,x])*dt*dx
D = np.sum(demand[t])
if P[t] > D_0*(Db[t]+Dmax):
Delta_P[t] = abs(P[t]-D_0*(Db[t]+Dmax))*dt
elif P[t] < D_0*(Db[t]+Dmin):
Delta_P[t] = abs(P[t]-D_0*(Db[t]+Dmin))*dt
else:
Delta_P[t] = abs(P[t]- D_0*(Db[t]+D))*dt
return (np.sum(Delta_u),np.sum(Delta_pi),np.sum(Delta_m),np.sum(Delta_P),np.sum(Delta_gamma))
Local transition version¶
tran_0 = np.array([0,1])
tran_interm = np.array([-1,0,1])
tran_n = np.array([-1,0])
def tran(x,n):
if x == 0:
return(tran_0)
if x == n-1:
return(tran_n)
else:
return(tran_interm)
def proj_Q(a_bar,b_bar,beta,n,lenY):
a = 0
b = np.zeros(lenY)
beta_bar = beta-b_bar
beta_bar_sort = np.sort(beta_bar)
k = 0
sum_beta_bar_sort = 0
derivative_beta = (k+1)*beta_bar_sort[k] - a_bar - sum_beta_bar_sort
while (derivative_beta < 0) and (k < lenY-1):
sum_beta_bar_sort = sum_beta_bar_sort + beta_bar_sort[k]
k = k+1
derivative_beta = (k+1)*beta_bar_sort[k] - a_bar - sum_beta_bar_sort
if (k==lenY-1) and (derivative_beta < 0):
sum_beta_bar_sort = sum_beta_bar_sort + beta_bar_sort[k]
k = lenY
a = (a_bar + sum_beta_bar_sort)/(k+1)
for y in range(lenY):
b[y] = np.min(np.array([b_bar[y],beta[y] - a]))
return (a,b)
def a_b_proj(P,gamma,u,m,w,T,n,r,alpha,beta):
dt=1/T
(new_a,new_b) = (np.zeros((T,n)),np.zeros((T-1,n,n)))
for t in range(T-1):
for x in range(n):
Y = tran(x,n) + x
c_1 = m[t,x]/r + u[t,x]/dt - gamma[t,x]
c_3 = w[t,x,Y]/r - alpha[t,x,Y]*P[t] - u[t+1,Y]/dt
(new_a[t,x],new_b[t,x,Y]) = proj_Q(c_1,c_3,beta[t,x,Y],n,len(Y))
return(new_a,new_b)
def u_argmin_L(P,gamma,a,b,m,w,m_0,alpha,T,n,r):
dt=1/T
new_u = np.zeros((T,n))
for t in range(T):
for x in range(n):
Y = tran(x,n) + x
s = len(Y)
if t==0:
new_u[t,x] = ((m_0[x] - m[t,x])/r + gamma[t,x] + a[t,x])*dt
elif t==(T-1):
new_u[t,x] = ((-m[t,x] + np.sum(w[t-1,Y,x]))/r + gamma[t,x] - np.sum(alpha[t-1,Y,x]*P[t-1] + b[t-1,Y,x]))/(1+s/dt)
else:
new_u[t,x] = ((-m[t,x] + np.sum(w[t-1,Y,x]))/r + gamma[t,x] + a[t,x] - np.sum(alpha[t-1,Y,x]*P[t-1] + b[t-1,Y,x]))*dt/(1+s)
return new_u
def alpha_bar(alpha,T,n):
new_alpha = np.zeros((T-1,n))
a = np.zeros(T-1)
for t in range(T-1):
for x in range(n):
Y = tran(x,n) + x
new_alpha[t,x] = np.sum(alpha[t,x,Y]**2)
a[t] = np.sum(new_alpha[t])
return(a)
def Local_P_prox_quad(u,b,w,n,r,alpha,var_alpha,Db,D_0,D_min,D_max,t):
dt=1/T
dx=1/n
Z = np.zeros((n,n))
for x in range(n):
Y = tran(x,n) + x
Z[x,Y] = (w[t,x,Y]/r - u[t+1,Y]/dt - b[t,x,Y])*alpha[t,x,Y]
X = np.sum(Z)/var_alpha[t]
lambde = 1/(r*var_alpha[t]*dx)
new_Pt = X - lambde*prox_phi_quad(X/lambde,Db[t],D_0,1/lambde,D_min,D_max)
return(new_Pt)
def m_w_gradient_step(P,gamma,u,a,b,m,w,q,T,n):
dt=1/T
new_m = np.zeros((T,n))
new_w = np.zeros((T-1,n,n))
for t in range(T-1):
for x in range(n):
Y = tran(x,n) + x
new_m[t,x] = m[t,x] + q*(u[t,x]/dt - gamma[t,x] - a[t,x])
new_w[t,x,Y] = w[t,x,Y] + q*(- alpha[t,x,Y]*P[t] - u[t+1,Y]/dt - b[t,x,Y])
new_m[T-1] = m[T-1] + q*(u[T-1] - gamma[T-1])
return (new_m,new_w)
def equilibrium_strategy(m,w,T,n,tol=1e-5):
strat = np.zeros((T-1,n,n))
for t in range(T-1):
for x in range(n):
Y = tran(x,n) + x
if m[t,x] > tol:
strat[t,x,Y] = w[t,x,Y]/m[t,x]
return(strat)
def d_p_mapping(m,w,gamma,P,alpha,beta,T,n,tol = 1e-3,mfg=1):
dt=1/T
dx=1/n
new_u = np.zeros((T,n))
new_pi = np.zeros((T-1,n,n))
f = np.zeros((T,n))
if mfg == 1:
f = gamma
new_u[T-1] = f[T-1]
for t in range(T-1):
for x in range(n):
cost = np.zeros(n)
Y = tran(x,n) + x
cost[Y] = beta[T-2-t,x,Y]*dt + alpha[T-2-t,x,Y]*P[T-2-t]*dt + new_u[T-1-t,Y]
argmin = np.argmin(cost[Y])
if m[T-2-t,x]*dx>tol:
new_pi[T-2-t,x,Y] = w[T-2-t,x,Y]/m[T-2-t,x]
else:
new_pi[T-2-t,x,Y[argmin]] = 1
new_u[T-2-t,x] = cost[Y[argmin]] + f[T-2-t,x]*dt
return(new_u,new_pi)
def verification(P,u,gamma,m,pi,w,T,n,beta,nu,m_0,alpha,Db,Dmin,Dmax,eta,mfg = 1):
cost = np.zeros((T-1,n))
Delta_u = np.zeros((T,n))
Delta_pi = np.zeros((T-1,n,n))
Delta_m = np.zeros((T,n))
Delta_gamma = np.zeros((T,n))
Delta_P = np.zeros(T-1)
demand = np.zeros((T-1,n,n))
dt=1/T
dx=1/n
f = np.zeros((T,n))
if mfg == 1:
f = gamma
Delta_m[0] = abs(m[0] - m_0)*dx
for x in range(n):
Delta_u[T-1,x] = abs(u[T-1,x] - f[T-1,x])*dx
for t in range(T-1):
for x in range(n):
Y = tran(x,n) + x
arg = beta[t,x,Y]*dt + alpha[t,x,Y]*P[t]*dt + u[t+1,Y]
demand[t,x,Y] = pi[t,x,Y]*m[t,x]*alpha[t,x,Y]*dx
cost[t,x] = np.sum(pi[t,x,Y]*(beta[t,x,Y]*dt + alpha[t,x,Y]*P[t]*dt)) + f[t,x]*dt
Delta_pi[t,x] = abs(np.sum(pi[t,x,Y]*arg) - np.sort(arg)[0])*dx
Delta_m[t+1,x] = abs(m[t+1,x] - np.sum(pi[t,Y,x]*m[t,Y]))*dx
Delta_u[t,x] = abs(u[t,x] - cost[t,x] - np.sum(pi[t,x,Y]*u[t+1,Y]))*dx
if gamma[t,x] < 0:
Delta_gamma[t,x] = abs(m[t,x])*dt*dx
elif gamma[t,x] > eta[t,x] + nu[t,x]:
Delta_gamma[t,x] = abs(m[t,x] - eta[t,x])*dt*dx
else:
Delta_gamma[t,x] = abs(gamma[t,x] - m[t,x])*dt*dx
D = np.sum(demand[t])
if P[t] > D_0*(Db[t]+Dmax):
Delta_P[t] = abs(D-Dmax)*dt
elif P[t] < D_0*(Db[t]+Dmin):
Delta_P[t] = abs(D-Dmin)*dt
else:
Delta_P[t] = abs(D- (P[t]/D_0 - Db[t]))*dt
return (np.sum(Delta_u),np.sum(Delta_pi),np.sum(Delta_m),np.sum(Delta_P),np.sum(Delta_gamma))
ADM-G¶
MFG¶
T = 50
n = T
alpha = np.zeros((T-1,n,n))
r = 2/n
q = r
xi = 1
var_alpha = alpha_bar(alpha,T,n)
m_0 = initial_mass(n)
beta = displacement_cost(T,n)
nu = np.zeros((T,n))
eta = sharp_penalisation_congestion(T,n)
Dmin = -10
Dmax = 10
D_0 = 1/2
Db = reference_demand(T)*0
P = np.zeros(T-1)
tilde_P = P
u = np.random.rand(T,n)
gamma = np.zeros((T,n))
m = np.random.rand(T,n) + 1
w = np.zeros((T-1,n,n)) + 1
(a,b) = (m,w)
N = 10000
start = time.time()
error = np.zeros(N)
mfg_error = np.zeros((N,5))
ev_m_s = np.zeros((N,T-1,n))
ev_m = np.zeros((N,T,n))
ev_u = np.zeros((N,T,n))
for i in range(N):
print(round(i/N*100, 2)," \r",end = '')
tilde_u = u_argmin_L(P,gamma,a,b,m,w,m_0,alpha,T,n,r)
tilde_gamma = Moreau_gamma_argmin_L(tilde_u,a,m,nu,eta,T,n,r)
(tilde_a,tilde_b) = a_b_proj(P,tilde_gamma,tilde_u,m,w,T,n,r,alpha,beta)
(tilde_m,tilde_w) = m_w_gradient_step(P,tilde_gamma,tilde_u,tilde_a,tilde_b,m,w,q,T,n)
(u,gamma,P,a,b,m,w) = GB_step(gamma,P,a,b,m,w,tilde_u,tilde_gamma,tilde_P,tilde_a,tilde_b,tilde_m,tilde_w,xi,alpha,var_alpha)
(U,Pi) = d_p_mapping(m,w,gamma,P,alpha,beta,T,n,tol = 1e-4)
mfg_error[i] = np.array(verification(P,U,gamma,m,Pi,w,T,n,beta,nu,m_0,alpha,Db,Dmin,Dmax,eta))
(ev_m_s[i],ev_m[i],ev_u[i]) = (mean_field_strategy(Pi,T,n),m,U)
end = time.time()
print("\n execution time :",round(end-start,2), "s")
99.99
execution time : 4777.45 s
Error Plot:
#plt.plot(np.log(mfg_error[:,0])/np.log(10), label = r'$\log(||\varepsilon_u||_1)$', color = 'blue')
plt.plot(np.log(mfg_error[:,1])/np.log(10), label = r'$\log(||\varepsilon_\pi||_1)$', color = 'orange')
plt.plot(np.log(mfg_error[:,2])/np.log(10), label = r'$\log(||\varepsilon_m||_1)$', color = 'purple')
plt.plot(np.log(mfg_error[:,4])/np.log(10), label = r'$\log(||\varepsilon_{\gamma}||_1)$', color = 'green')
#plt.plot(np.log(mfg_error[:,3])/np.log(10), label = r'$\log(||\varepsilon_P||_1)$', color = 'red')
plt.xlabel('iteration')
plt.ylabel(r'$\log(||\varepsilon||)$')
plt.legend()
plt.savefig('ADMG_constraint_mfg_error.png', dpi=500, bbox_inches='tight')
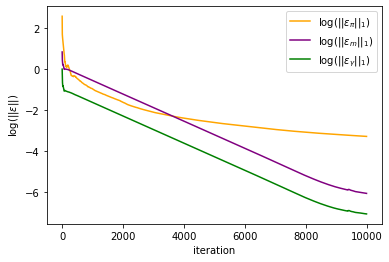
t = np.linspace(0, 1, T)
y = np.linspace(0, 1, n)
X, Y = np.meshgrid(y, t)
Z = eta
fig = plt.figure()
ax = plt.axes(projection='3d')
#ax.contour3D(X, Y, Z, 50, cmap='binary')
ax.plot_surface(X, Y, Z, rstride=1, cstride=1, cmap='Greys', edgecolor='none')
#ax.plot_wireframe(X, Y, Z, rstride=1, cstride=1, cmap='viridis', edgecolor='none')
ax.set_xlabel('state')
ax.set_ylabel('time')
ax.set_zlabel('penalisation')
plt.savefig('eta.png', dpi=500, bbox_inches='tight')
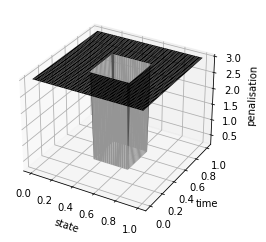
#%matplotlib notebook
Z = m
fig = plt.figure()
ax = plt.axes(projection='3d')
#ax.contour3D(X, Y, Z, 50, cmap='binary')
ax.plot_surface(X, Y, Z, rstride=1, cstride=1, cmap='YlOrRd', edgecolor='none')
#ax.plot_wireframe(X, Y, Z, rstride=1, cstride=1, cmap='v£iridis', edgecolor='none')
ax.set_xlabel('state')
ax.set_ylabel('time')
ax.set_zlabel('m')
plt.savefig('ADMG_constraint_mfg_m.png', dpi=500, bbox_inches='tight')
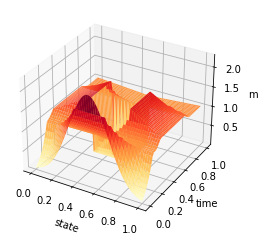
X, Y = np.meshgrid(t, y)
Z = m
minm = np.min(Z)
maxm = np.max(Z)
levels = np.linspace(minm,maxm,10)
fig, ax = plt.subplots()
im = ax.imshow(Z, interpolation='bilinear', origin='lower',
cmap='YlOrRd')
plt.axis('off')
CS = ax.contour(Z,levels, origin='lower', colors='black',
linewidths=1)
CBI = fig.colorbar(im)
plt.savefig('ADMG_constraint_mfg_m_contour.png', dpi=500, bbox_inches='tight')
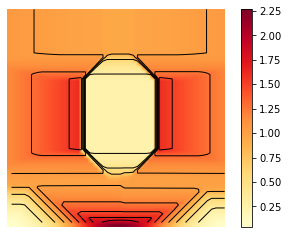
t = np.linspace(0, 1, T)
y = np.linspace(0, 1, n)
Nb_im = 2000
X, Y = np.meshgrid(y, t)
Z = ev_m[:Nb_im]
def update_plot(frame_number, zarray, plot):
plot[0].remove()
plt.title('Iteration :%s'%frame_number)
plot[0] = ax.plot_surface(X, Y, Z[frame_number,:,:], cmap="YlOrRd",edgecolor='none')
fig = plt.figure()
ax = fig.add_subplot(111, projection='3d')
fps = 100
plot = [ax.plot_surface(X, Y, Z[0,:,:], rstride=1, cstride=1)]
ani = animation.FuncAnimation(fig, update_plot, Nb_im, fargs=(Z, plot), interval=2000/fps)
ani.save('ADMG_constraint_mfg_m_convergence.mp4',writer='ffmpeg',fps=fps)
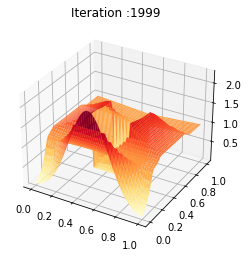
Z = U
fig = plt.figure()
ax = plt.axes(projection='3d')
#ax.contour3D(X, Y, Z, 50, cmap='magma')
ax.plot_surface(X, Y, Z, rstride=1, cstride=1, cmap='viridis', edgecolor='none')
#ax.plot_wireframe(X, Y, Z, rstride=1, cstride=1, cmap='viridis', edgecolor='none')
ax.set_xlabel('state')
ax.set_ylabel('time')
ax.set_zlabel('value')
plt.savefig('ADMG_constraint_mfg_u.png', dpi=500, bbox_inches='tight')
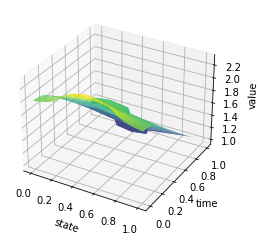
X, Y = np.meshgrid(t, y)
Z = U
minu = np.min(Z)
maxu = np.max(Z)
levels = np.linspace(minu,maxu,10)
fig, ax = plt.subplots()
im = ax.imshow(Z, interpolation='bilinear', origin='lower',
cmap='viridis')
plt.axis('off')
CS = ax.contour(Z,levels, origin='lower', colors='black',
linewidths=1)
CBI = fig.colorbar(im)
plt.savefig('ADMG_constraint_mfg_u_contour.png', dpi=500, bbox_inches='tight')
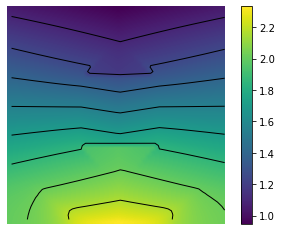
t = np.linspace(0, 1, T)
y = np.linspace(0, 1, n)
Nb_im = 2000
X, Y = np.meshgrid(y, t)
Z = ev_u[:Nb_im]
def update_plot(frame_number, zarray, plot):
plot[0].remove()
plt.title('Iteration :%s'%frame_number)
plot[0] = ax.plot_surface(X, Y, Z[frame_number,:,:], cmap="viridis",edgecolor='none')
fig = plt.figure()
ax = fig.add_subplot(111, projection='3d')
fps = 100
plot = [ax.plot_surface(X, Y, Z[0,:,:], rstride=1, cstride=1)]
ani = animation.FuncAnimation(fig, update_plot, Nb_im, fargs=(Z, plot), interval=2000/fps)
ani.save('ADMG_constraint_mfg_u_convergence.mp4',writer='ffmpeg',fps=fps)
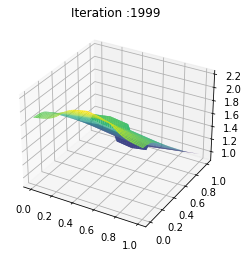
Z = gamma
fig = plt.figure()
ax = plt.axes(projection='3d')
#ax.contour3D(X, Y, Z, 50, cmap='binary')
ax.plot_surface(X, Y, Z, rstride=1, cstride=1, cmap='YlOrRd', edgecolor='none')
#ax.plot_wireframe(X, Y, Z, rstride=1, cstride=1, cmap='viridis', edgecolor='none')
ax.set_xlabel('state')
ax.set_ylabel('time')
ax.set_zlabel('gamma')
plt.savefig('ADMG_constraint_mfg_gamma.png', dpi=500, bbox_inches='tight')
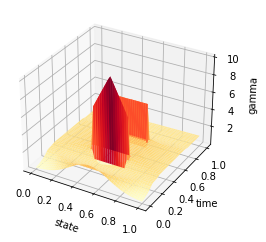
ti = np.linspace(0, 1, T-1)
y = np.linspace(0, 1, n)
X, Y = np.meshgrid(y, ti)
Z = mean_field_strategy(Pi,T,n)
fig = plt.figure()
ax = plt.axes(projection='3d')
#ax.contour3D(X, Y, Z, 50, cmap='magma')
ax.plot_surface(X, Y, Z, rstride=1, cstride=1, cmap='inferno', edgecolor='none')
#ax.plot_wireframe(X, Y, Z, rstride=1, cstride=1, cmap='viridis', edgecolor='none')
ax.set_xlabel('state')
ax.set_ylabel('time')
ax.set_zlabel('mean strategy')
plt.savefig('ADMG_constraint_mfg_mean_strategy.png', dpi=500, bbox_inches='tight')
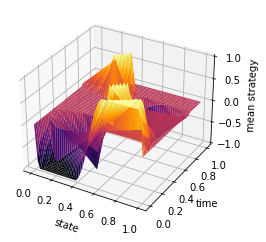
minm = np.min(Z)
maxm = np.max(Z)
levels = np.linspace(minm,maxm,10)
fig, ax = plt.subplots()
im = ax.imshow(Z, interpolation='bilinear', origin='lower',
cmap='inferno')
plt.axis('off')
CS = ax.contour(Z,levels, origin='lower', colors='black',
linewidths=1)
CBI = fig.colorbar(im)
plt.savefig('ADMG_constraint_mfg_mean_strategy_contour.png', dpi=500, bbox_inches='tight')
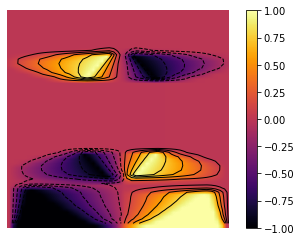
ti = np.linspace(0, 1, T-1)
y = np.linspace(0, 1, n)
Nb_im = 2000
X, Y = np.meshgrid(y, ti)
Z = ev_m_s[:Nb_im]
def update_plot(frame_number, zarray, plot):
plot[0].remove()
plt.title('Iteration :%s'%frame_number)
plot[0] = ax.plot_surface(X, Y, Z[frame_number,:,:], cmap="inferno",edgecolor='none')
fig = plt.figure()
ax = fig.add_subplot(111, projection='3d')
fps = 100
plot = [ax.plot_surface(X, Y, Z[0,:,:], rstride=1, cstride=1)]
ani = animation.FuncAnimation(fig, update_plot, Nb_im, fargs=(Z, plot), interval=2000/fps)
ani.save('ADMG_constraint_mfg_mean_strategy_convergence.mp4',writer='ffmpeg',fps=fps)
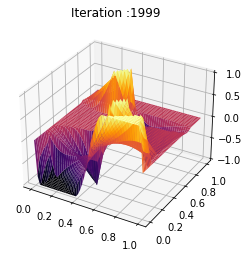
MFGC¶
T = 50
n = T
alpha = alpha_cost(T,n)
r = 1/2
q = r
xi = 1
var_alpha = alpha_bar(alpha,T,n)
m_0 = initial_mass(n)
beta = displacement_cost(T,n)
nu = np.zeros((T,n))
D_0 = 1/2
Db = reference_demand(T)*2
Dmin = -2
Dmax = 0
P = np.zeros(T-1)
u = np.random.rand(T,n)
gamma = np.zeros((T,n))
tilde_gamma = gamma
m = np.random.rand(T,n)
w = np.zeros((T-1,n,n)) + 1
(a,b) = (m,w)
N = 10000
start = time.time()
error = np.zeros(N)
ev_m_s = np.zeros((N,T-1,n))
ev_m = np.zeros((N,T,n))
ev_u = np.zeros((N,T,n))
mfg_error = np.zeros((N,5))
for i in range(N):
print(round(i/N*100, 2)," \r",end = '')
tilde_u = u_argmin_L(P,gamma,a,b,m,w,m_0,alpha,T,n,r)
tilde_P = P_argmin_L(tilde_u,b,w,T,n,r,alpha,var_alpha,Db,D_0,Dmin,Dmax)
(tilde_a,tilde_b) = a_b_proj(tilde_P,tilde_gamma,tilde_u,m,w,T,n,r,alpha,beta)
(tilde_m,tilde_w) = m_w_gradient_step(tilde_P,tilde_gamma,tilde_u,tilde_a,tilde_b,m,w,q,T,n)
(u,gamma,P,a,b,m,w) = GB_step(gamma,P,a,b,m,w,tilde_u,tilde_gamma,tilde_P,tilde_a,tilde_b,tilde_m,tilde_w,xi,alpha,var_alpha,mfg=0)
(U,Pi) = d_p_mapping(m,w,gamma,P,alpha,beta,T,n,tol = 1e-4,mfg = 0)
mfg_error[i] = np.array(verification(P,U,gamma,m,Pi,w,T,n,beta,nu,m_0,alpha,Db,Dmin,Dmax,eta,mfg=0))
(ev_m_s[i],ev_m[i],ev_u[i]) = (mean_field_strategy(Pi,T,n),m,U)
end = time.time()
print("\n execution time :",round(end-start,2), "s")
99.99
execution time : 5060.99 s
Error Plot:
#plt.plot(np.log(mfg_error[:,0])/np.log(10), label = r'$\log(||\varepsilon_u||_1)$', color = 'blue')
plt.plot(np.log(mfg_error[:,1])/np.log(10), label = r'$\log(||\varepsilon_\pi||_1)$', color = 'orange')
plt.plot(np.log(mfg_error[:,2])/np.log(10), label = r'$\log(||\varepsilon_m||_1)$', color = 'purple')
#plt.plot(np.log(mfg_error[:,4])/np.log(10), label = r'$\log(||\varepsilon_{\gamma}||_1)$', color = 'green')
plt.plot(np.log(mfg_error[:,3])/np.log(10), label = r'$\log(||\varepsilon_P||_1)$', color = 'red')
plt.xlabel('iteration')
plt.ylabel(r'$\log(||\varepsilon||_1)$')
plt.legend()
plt.savefig('ADMG_constraint_mfgc_error.png', dpi=500, bbox_inches='tight')
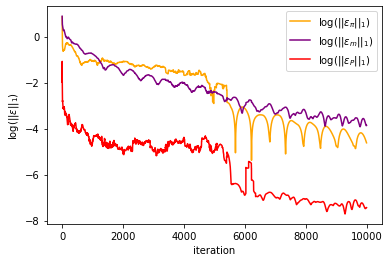
Outputs
t = np.linspace(0, 1, T)
y = np.linspace(0, 1, n)
X, Y = np.meshgrid(t, y)
Z = m
fig = plt.figure()
ax = plt.axes(projection='3d')
#ax.contour3D(X, Y, Z, 50, cmap='binary')
ax.plot_surface(X, Y, Z, rstride=1, cstride=1, cmap='YlOrRd', edgecolor='none')
#ax.plot_wireframe(X, Y, Z, rstride=1, cstride=1, cmap='v£iridis', edgecolor='none')
ax.set_xlabel('state')
ax.set_ylabel('time')
ax.set_zlabel('m')
plt.savefig('ADMG_constraint_mfgc_m.png', dpi=500, bbox_inches='tight')
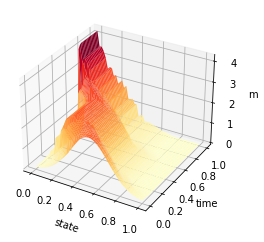
Z = m
minm = np.min(Z)
maxm = np.max(Z)
levels = np.linspace(minm,maxm,10)
fig, ax = plt.subplots()
im = ax.imshow(Z, interpolation='bilinear', origin='lower',
cmap='YlOrRd')
plt.axis('off')
CS = ax.contour(Z,levels, origin='lower', colors='black',
linewidths=1)
CBI = fig.colorbar(im)
plt.savefig('ADMG_constraint_mfgc_m_contour.png', dpi=500, bbox_inches='tight')
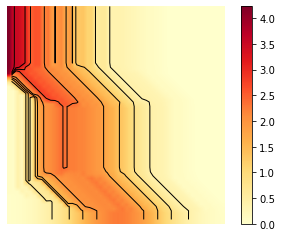
t = np.linspace(0, 1, T)
y = np.linspace(0, 1, n)
Nb_im = 2000
X, Y = np.meshgrid(y, t)
Z = ev_m[:Nb_im]
def update_plot(frame_number, zarray, plot):
plot[0].remove()
plt.title('Iteration :%s'%frame_number)
plot[0] = ax.plot_surface(X, Y, Z[frame_number,:,:], cmap="YlOrRd",edgecolor='none')
fig = plt.figure()
ax = fig.add_subplot(111, projection='3d')
fps = 100
plot = [ax.plot_surface(X, Y, Z[0,:,:], rstride=1, cstride=1)]
ani = animation.FuncAnimation(fig, update_plot, Nb_im, fargs=(Z, plot), interval=2000/fps)
ani.save('ADMG_constraint_mfgc_m_convergence.mp4',writer='ffmpeg',fps=fps)
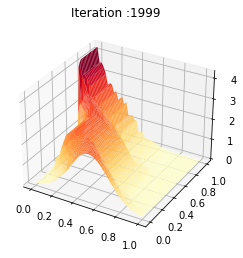
Z = U
fig = plt.figure()
ax = plt.axes(projection='3d')
#ax.contour3D(X, Y, Z, 50, cmap='magma')
ax.plot_surface(X, Y, Z, rstride=1, cstride=1, cmap='viridis', edgecolor='none')
#ax.plot_wireframe(X, Y, Z, rstride=1, cstride=1, cmap='viridis', edgecolor='none')
ax.set_xlabel('state')
ax.set_ylabel('time')
ax.set_zlabel('value')
plt.savefig('ADMG_constraint_mfgc_u.png', dpi=500, bbox_inches='tight')
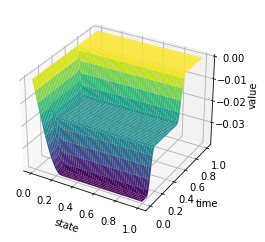
X, Y = np.meshgrid(t, y)
Z = U
minu = np.min(Z)
maxu = np.max(Z)
levels = np.linspace(minu,maxu,10)
fig, ax = plt.subplots()
im = ax.imshow(Z, interpolation='bilinear', origin='lower',
cmap='viridis')
plt.axis('off')
CS = ax.contour(Z,levels, origin='lower', colors='black',
linewidths=1)
CBI = fig.colorbar(im)
plt.savefig('ADMG_constraint_mfgc_u_contour.png', dpi=500, bbox_inches='tight')
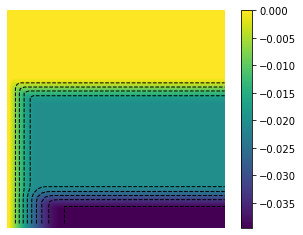
t = np.linspace(0, 1, T)
y = np.linspace(0, 1, n)
Nb_im = 2000
X, Y = np.meshgrid(y, t)
Z = ev_u[:Nb_im]
def update_plot(frame_number, zarray, plot):
plot[0].remove()
plt.title('Iteration :%s'%frame_number)
plot[0] = ax.plot_surface(X, Y, Z[frame_number,:,:], cmap="viridis",edgecolor='none')
fig = plt.figure()
ax = fig.add_subplot(111, projection='3d')
fps = 100
plot = [ax.plot_surface(X, Y, Z[0,:,:], rstride=1, cstride=1)]
ani = animation.FuncAnimation(fig, update_plot, Nb_im, fargs=(Z, plot), interval=2000/fps)
ani.save('ADMG_constraint_mfgc_u_convergence.mp4',writer='ffmpeg',fps=fps)
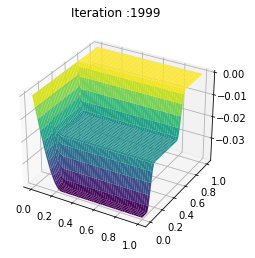
ti = np.linspace(0, 1, T-1)
y = np.linspace(0, 1, n)
X, Y = np.meshgrid(y, ti)
Z = mean_field_strategy(Pi,T,n)
fig = plt.figure()
ax = plt.axes(projection='3d')
#ax.contour3D(X, Y, Z, 50, cmap='magma')
ax.plot_surface(X, Y, Z, rstride=1, cstride=1, cmap='inferno', edgecolor='none')
#ax.plot_wireframe(X, Y, Z, rstride=1, cstride=1, cmap='viridis', edgecolor='none')
ax.set_xlabel('state')
ax.set_ylabel('time')
ax.set_zlabel('mean strategy')
plt.savefig('ADMG_constraint_mfgc_mean_strategy.png', dpi=500, bbox_inches='tight')
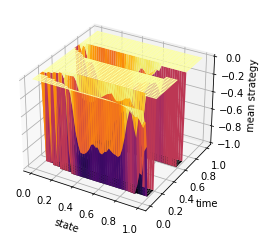
minm = np.min(Z)
maxm = np.max(Z)
levels = np.linspace(minm,maxm,10)
fig, ax = plt.subplots()
im = ax.imshow(Z, interpolation='bilinear', origin='lower',
cmap='inferno')
plt.axis('off')
CS = ax.contour(Z,levels, origin='lower', colors='black',
linewidths=1)
CBI = fig.colorbar(im)
plt.savefig('ADMG_constraint_mfgc_mean_strategy_contour.png', dpi=500, bbox_inches='tight')
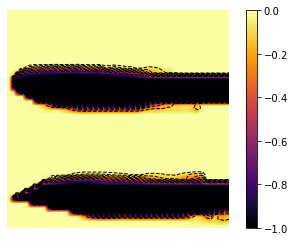
ti = np.linspace(0, 1, T-1)
y = np.linspace(0, 1, n)
Nb_im = 2000
X, Y = np.meshgrid(y, ti)
Z = ev_m_s[:Nb_im]
def update_plot(frame_number, zarray, plot):
plot[0].remove()
plt.title('Iteration :%s'%frame_number)
plot[0] = ax.plot_surface(X, Y, Z[frame_number,:,:], cmap="inferno",edgecolor='none')
fig = plt.figure()
ax = fig.add_subplot(111, projection='3d')
fps = 100
plot = [ax.plot_surface(X, Y, Z[0,:,:], rstride=1, cstride=1)]
ani = animation.FuncAnimation(fig, update_plot, Nb_im, fargs=(Z, plot), interval=2000/fps)
ani.save('ADMG_constraint_mfgc_mean_strategy_convergence.mp4',writer='ffmpeg',fps=fps)
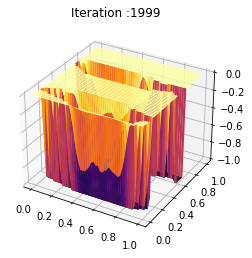
def real_demand(Db,D_0,alpha,w,T,n):
dx=1/n
D_eff = np.zeros(T-1)
for t in range(T-1):
D_eff[t] = (np.sum(alpha[t]*w[t])*dx + Db[t])
return(D_eff)
plt.plot(np.linspace(0,1,T-1),real_demand(Db,D_0,alpha,w,T,n), 'r-',label='D_eff')
plt.plot(np.linspace(0,1,T-1),Db, color = 'dimgrey', label = r'$\bar{D}$')
plt.legend(loc='upper right',
ncol=2)
plt.xlabel('time')
plt.ylabel('Demand')
plt.savefig('ADMG_constraint_mfgc_D.png', dpi=500, bbox_inches='tight')
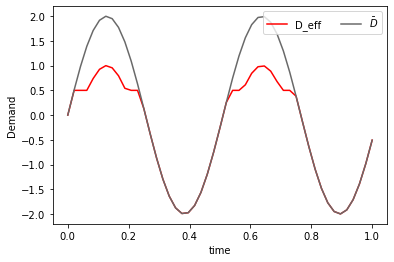
plt.plot(np.linspace(0,1,T-1),P, 'r-',label='Price')
plt.legend(loc='upper right',
ncol=2)
plt.xlabel('time')
plt.ylabel('Price')
plt.savefig('ADMG_constraint_mfgc_P.png', dpi=500, bbox_inches='tight')
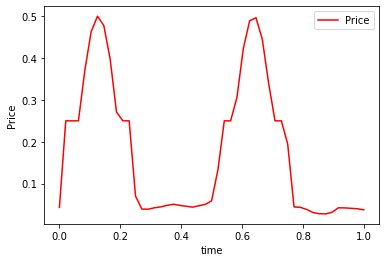
- HTY12
Bingsheng He, Min Tao, and Xiaoming Yuan. Alternating direction method with gaussian back substitution for separable convex programming. SIAM Journal on Optimization, 22(2):313–340, 2012.